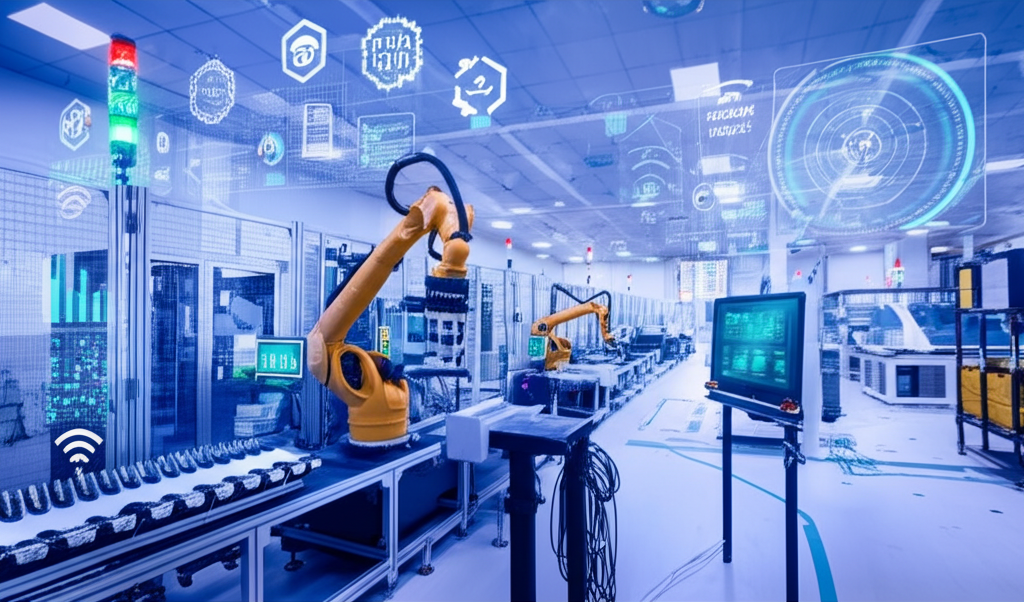
Edge AI: Powering the Next Generation of IoT Solutions
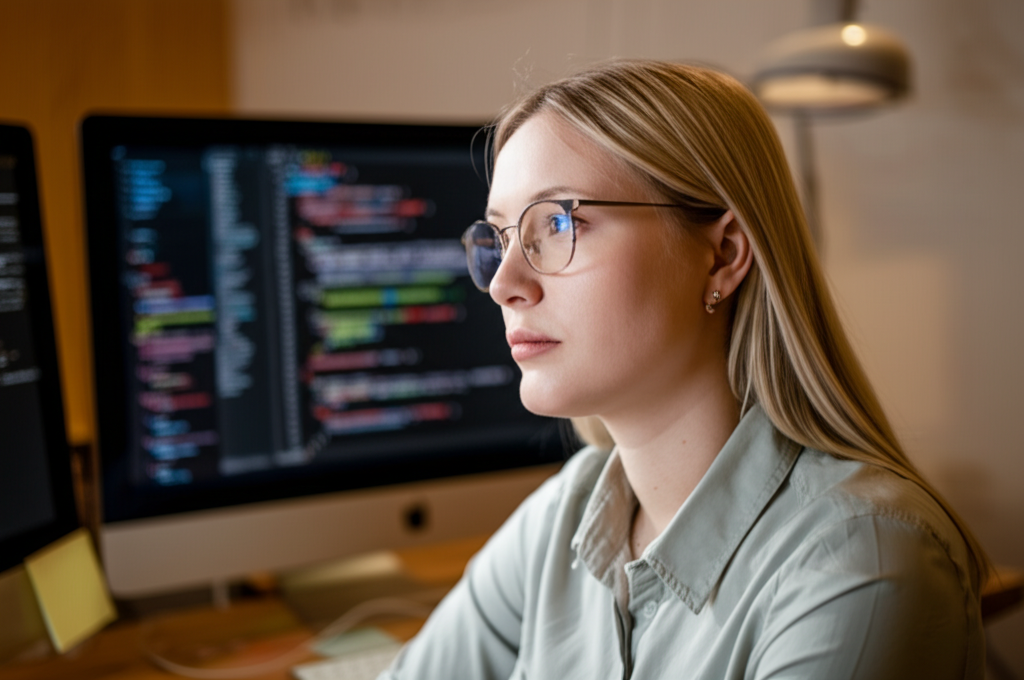
Edge AI: Powering the Next Generation of IoT Solutions
The convergence of edge computing and artificial intelligence is revolutionizing the Internet of Things (IoT) landscape. Edge AI—the deployment of AI algorithms directly on devices or local edge servers rather than in the cloud—is enabling a new generation of IoT solutions with enhanced capabilities, improved efficiency, and better privacy protections. This shift is creating significant opportunities for businesses across industries.
The Evolution from Cloud to Edge
Traditional IoT architectures have relied heavily on cloud computing, with devices sending data to centralized servers for processing and analysis. While effective for many applications, this approach has limitations:
- Latency: The time required to send data to the cloud and receive a response can be problematic for time-sensitive applications.
- Bandwidth consumption: Transmitting large volumes of raw data is costly and inefficient.
- Connectivity requirements: Dependence on reliable internet connections creates vulnerabilities.
- Privacy concerns: Sending potentially sensitive data to the cloud raises security and compliance issues.
- Energy efficiency: Constant data transmission consumes significant power, limiting battery life for IoT devices.
Key Benefits of Edge AI
Edge AI addresses these challenges by processing data locally, offering several advantages:
- Real-time processing: Enabling immediate responses for time-critical applications without cloud roundtrips.
- Reduced bandwidth: Sending only relevant insights rather than raw data significantly decreases network traffic.
- Enhanced privacy: Keeping sensitive data local rather than transmitting it to the cloud.
- Operational reliability: Maintaining functionality even when cloud connectivity is limited or unavailable.
- Extended battery life: Minimizing power-intensive data transmissions for resource-constrained devices.
Transformative Applications Across Industries
Edge AI is enabling innovative IoT applications in numerous sectors:
Manufacturing
- Predictive maintenance: Real-time analysis of equipment performance to predict failures before they occur.
- Quality control: Visual inspection systems that can identify defects instantly on production lines.
- Worker safety: Monitoring for dangerous situations and providing immediate alerts.
- Process optimization: Continuous analysis and adjustment of manufacturing parameters for maximum efficiency.
Healthcare
- Remote patient monitoring: Smart devices that can detect anomalies and alert healthcare providers without constantly streaming sensitive health data.
- Fall detection: Wearables that can identify falls and automatically summon help.
- Medication adherence: Systems that remind and verify patients are taking medications correctly.
- Disease detection: Devices that can identify early warning signs of conditions like respiratory diseases or cardiac issues.
Smart Cities
- Traffic management: Intelligent traffic lights that adapt to real-time conditions without central coordination.
- Public safety: Surveillance systems that can identify potential security issues while preserving privacy.
- Environmental monitoring: Sensors that can detect air quality issues, flooding risks, or other hazards and trigger immediate responses.
- Infrastructure maintenance: Monitoring systems that can identify developing problems in bridges, roads, and utilities.
Retail
- Inventory management: Smart shelves that track stock levels and detect misplaced items in real-time.
- Customer analytics: Privacy-preserving foot traffic analysis that provides insights without identifying individuals.
- Automated checkout: Systems that enable frictionless shopping experiences without constant cloud connectivity.
- Loss prevention: Intelligent systems that can detect potential theft while minimizing false alarms.
Implementation Challenges
Despite its benefits, implementing Edge AI presents several challenges:
- Hardware constraints: Deploying sophisticated AI models on devices with limited processing power, memory, and energy.
- Model optimization: Adapting AI algorithms to function efficiently within edge constraints without sacrificing accuracy.
- Deployment complexity: Managing and updating AI models across distributed edge devices.
- Heterogeneous environments: Supporting diverse device types, operating systems, and capabilities.
- Security concerns: Protecting edge devices from physical tampering and cyber attacks.
Emerging Solutions and Best Practices
Several approaches are helping organizations overcome these challenges:
- Model compression techniques: Methods like quantization, pruning, and knowledge distillation to create smaller, more efficient AI models.
- Specialized edge AI hardware: New processors designed specifically for AI workloads at the edge.
- Hybrid edge-cloud architectures: Balanced approaches that leverage both edge processing and cloud capabilities.
- Edge MLOps: Adapted DevOps practices for managing machine learning models on edge devices.
- Federated learning: Training AI models across distributed devices without centralizing sensitive data.
Conclusion
Edge AI represents a fundamental shift in how IoT systems are designed and deployed. By bringing intelligence directly to where data is generated, organizations can create more responsive, efficient, and privacy-preserving solutions. While challenges remain, rapid advances in hardware, software, and implementation methodologies are making Edge AI increasingly accessible. Organizations that begin exploring and implementing Edge AI strategies now will be well-positioned to lead the next generation of IoT innovation in their industries.